Client: PETRA Data Science
Date: 2020-2024
Type:UX, UI, SaaS Product
For project geologists and engineers, ROCKRay is a machine learning solution that provides a low cost, timely and effective way of predicting rock properties along sections of drill core that have not undergone laboratory characterisation.
ROCKRay digitally merges measured rock properties with core data, to predict and project these rock properties along untested lengths of core using machine learning. This case study shows a particular feature of how to update machine learning models on a self-service platform.
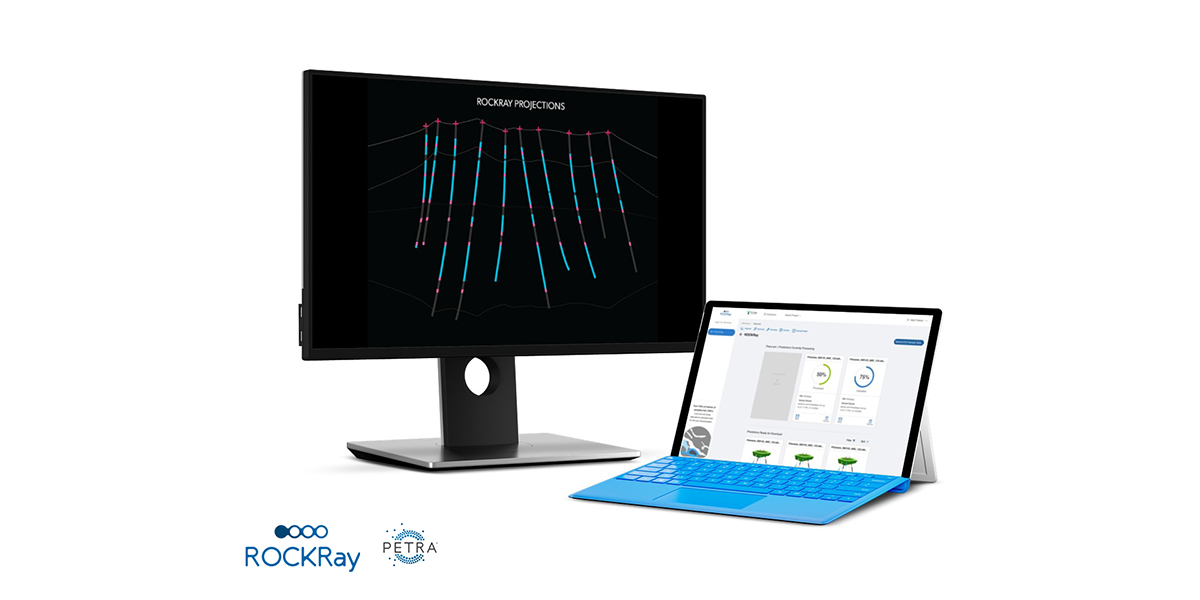
Self service data update platform
The Challenge
For a user, updating existing machine learning models is confusing and often times a very manual process.
Find a way that makes the data fusion/ model retrain process friendlier and logical – make it more DIY.
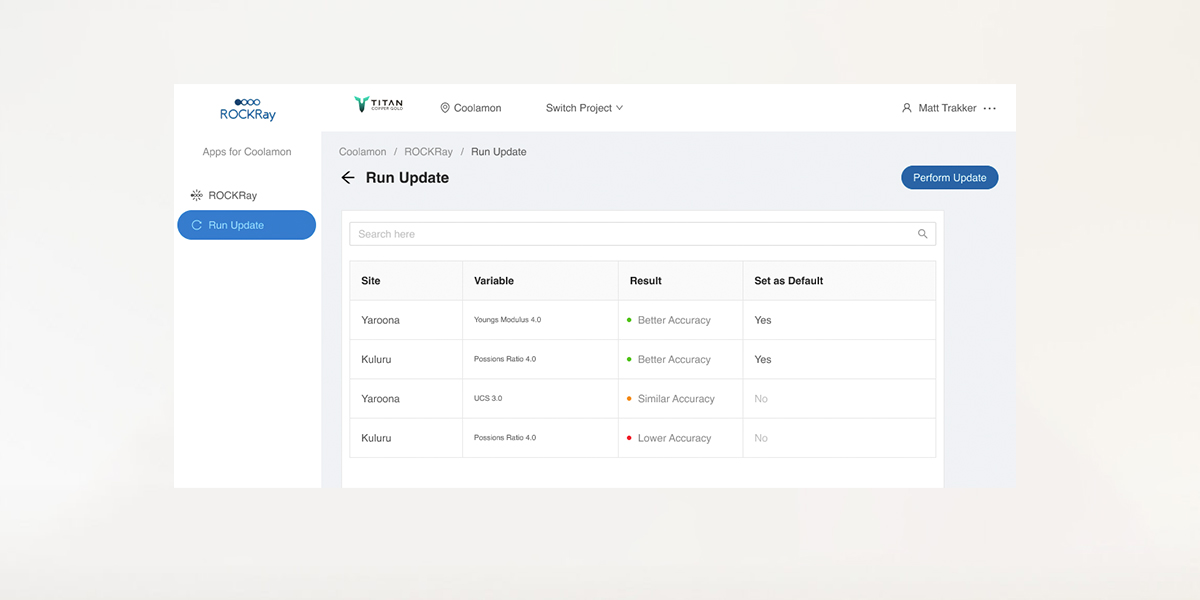
Believing there is a solution
Hypothesis
We believed that giving the user the ability to control the machine learning updates themselves would give them more insight and transparency on the data which in turn would turn into a higher trust score.
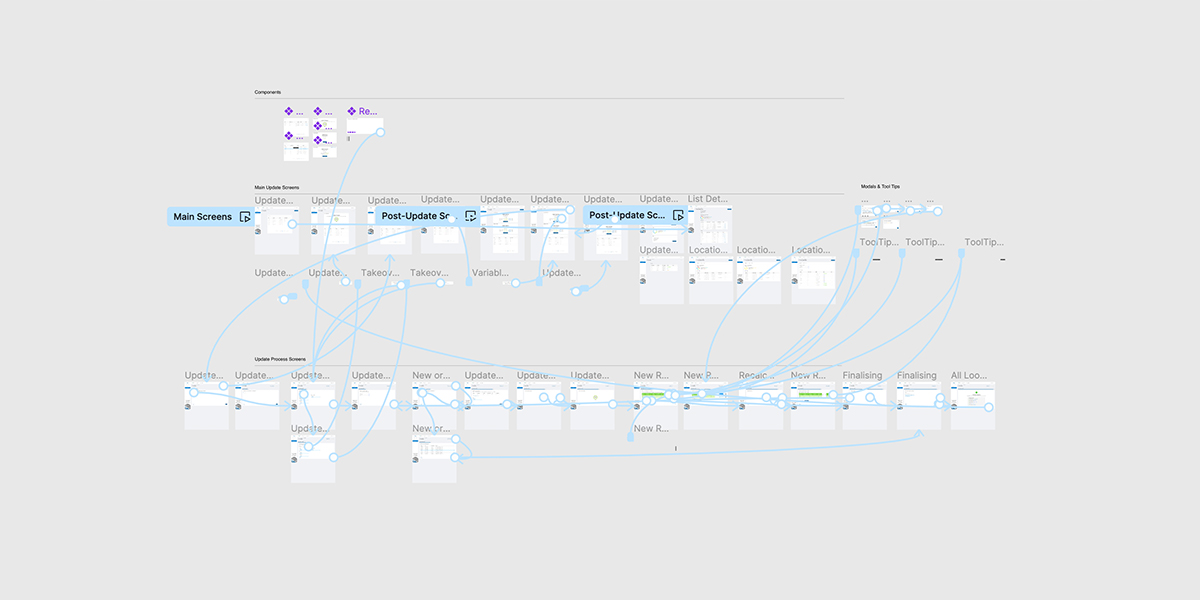
It is plausible?
Scoping the solution
This was a purely internal prototyping and testing sprint where I took into account hypothetical user stories (devised by our team and SME’s) and came up with a solution to use as part of research.
We discussed the hypothesis with our internal team and then I moved to wireframing to identify issues like clunkiness early on.
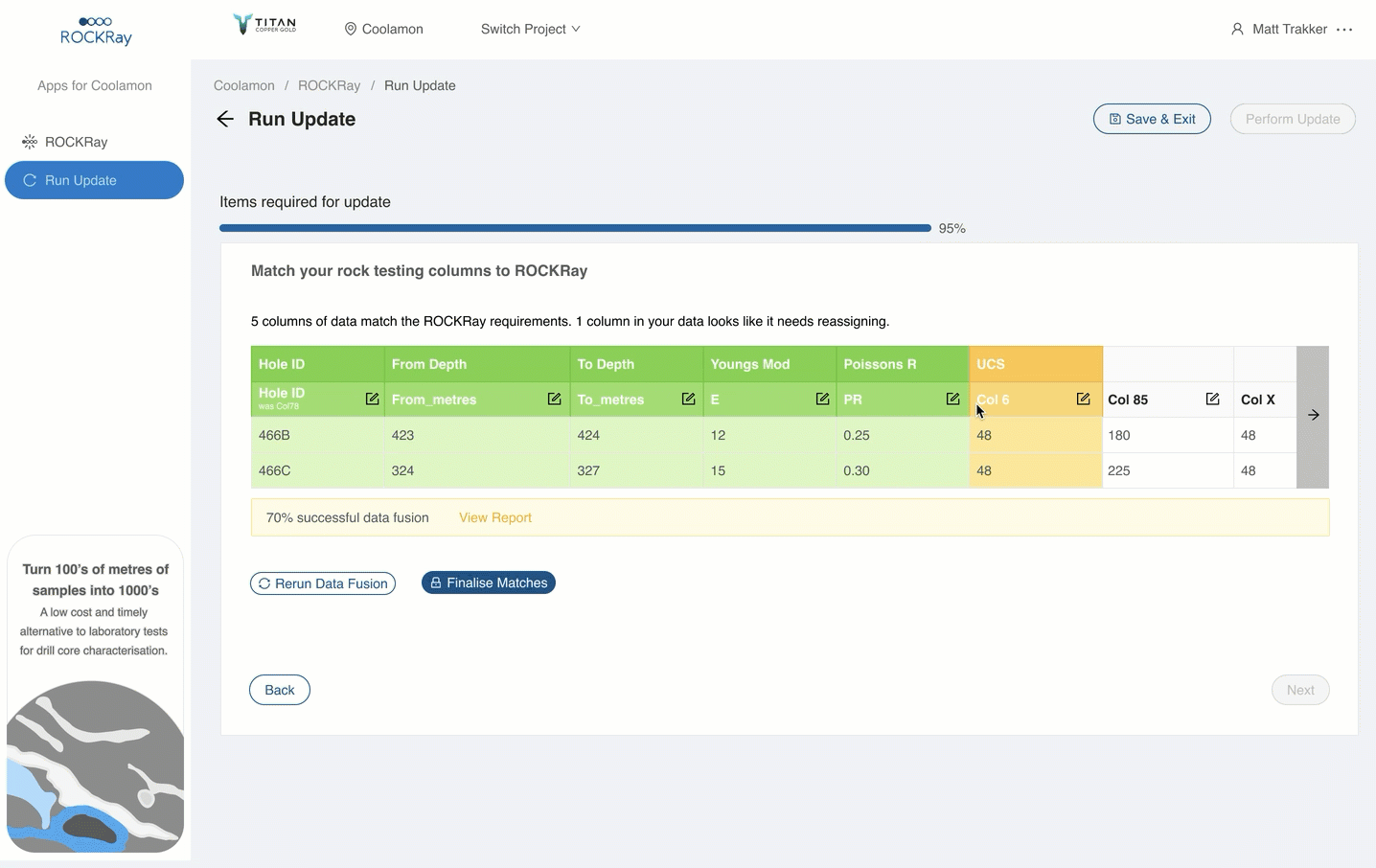
Creating & testing
Protoyping
Once I had established a few iterations, I presented the clickable prototypes to the team for initial testing and feedback.
This proved to be extremely useful as we could both discuss limitations and possibilites freely.
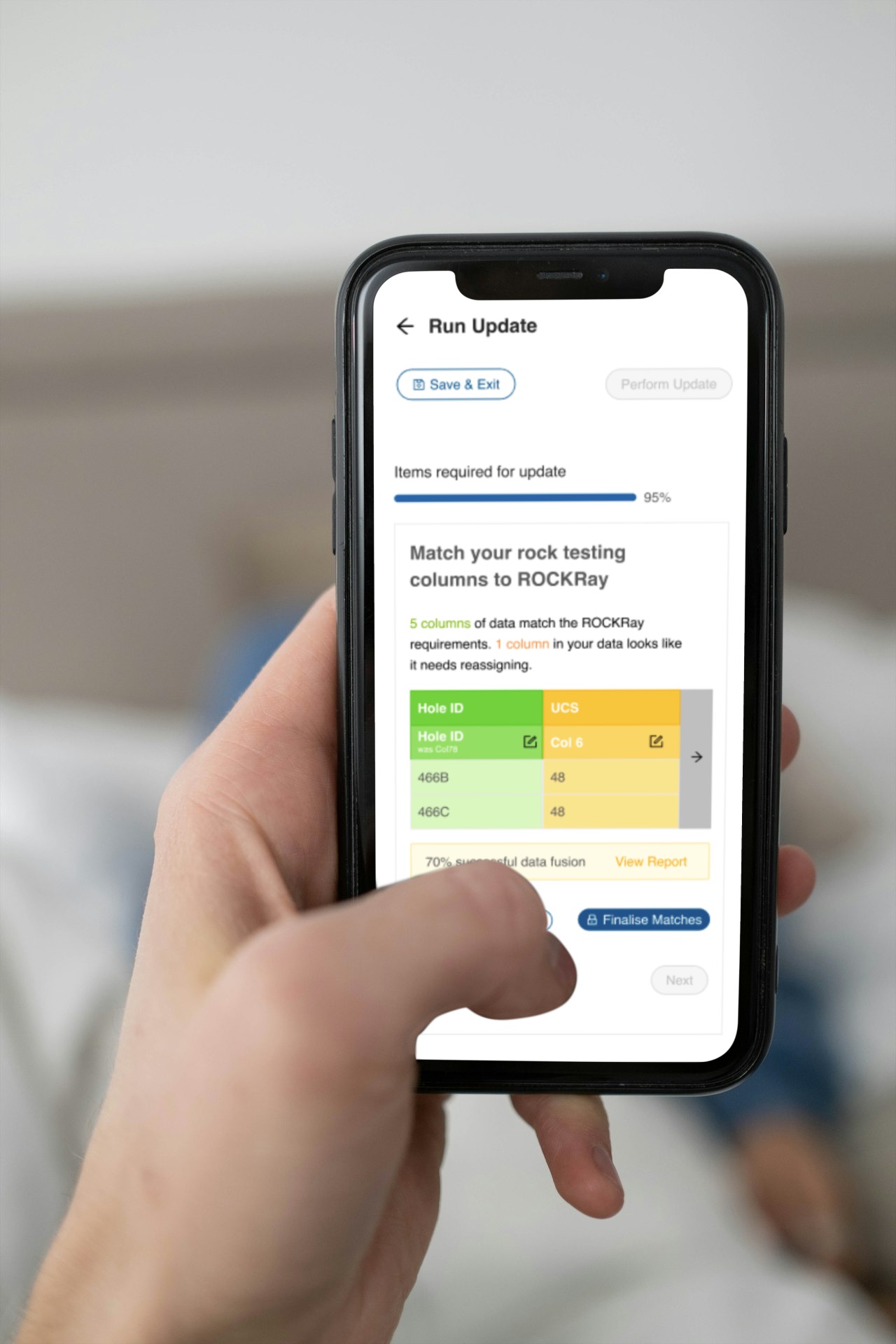
Creating tangible product designs
Implementation
From my Figma high res prototype, the team was able to build a proof of concept and we have been testing it with key stakeholders which include our customers.
So far the customer still believes the concept is a bit confusing as there is a lot of terminology they are not familiar with, so this presents itself as an opporunity to iterate and perhaps conduct more research on users understandings of machine learning.
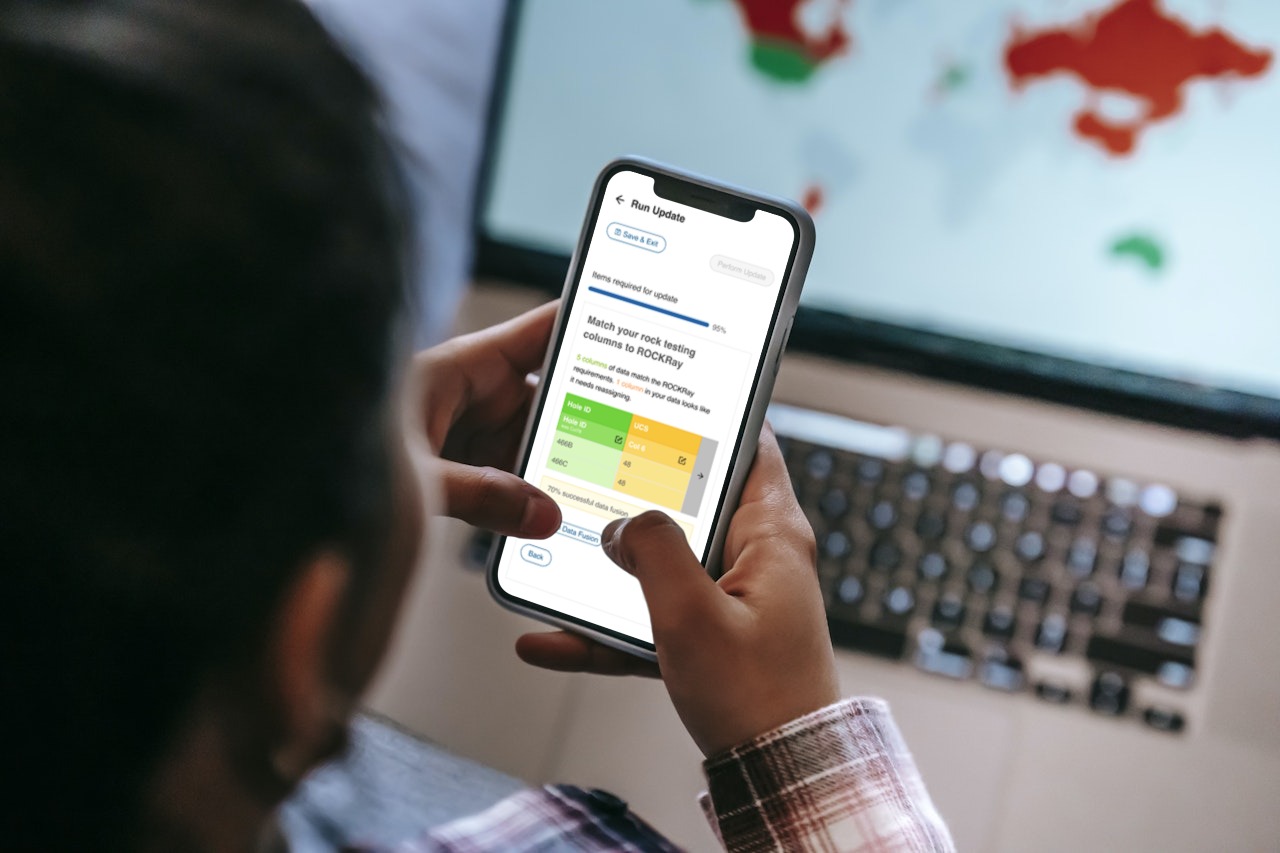
Significant behavioural acceptance
The Results
At this stage we have strong stakeholder interest and look to be deploying this feature once we nail the usability.